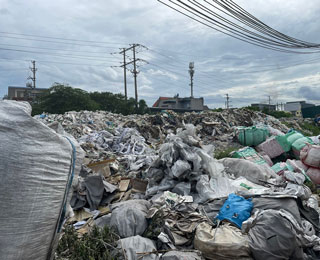
Co-processing potential for the Vietnamese cement industry
Vietnam’s cement industry accounts for 17 per cent of the country’s fossil fuel-derived CO 2 em...
At the Pennsuco plant in Medley, Florida, advanced AI-driven insights are reshaping cement production through predictive maintenance and process optimisation, crucial for enhancing operational efficiency in today’s competitive cement industry. By leveraging real-time sensor data across plant operations, this technology is significantly reducing downtime and streamlining processes. This case study highlights how AI integration can not only improve operational control but also support sustainability goals, illustrating the transformative potential of digital solutions in modern cement manufacturing. by CemAI, USA
Subscribe to get access to all News, Articles and Video sections of the CemNet.com website.
SubscribeOur editors pick the top news delivered to your inbox each day.